Mon Apr 11 2022
How Artificial Intelligence is Shaping the Future of Test Automation
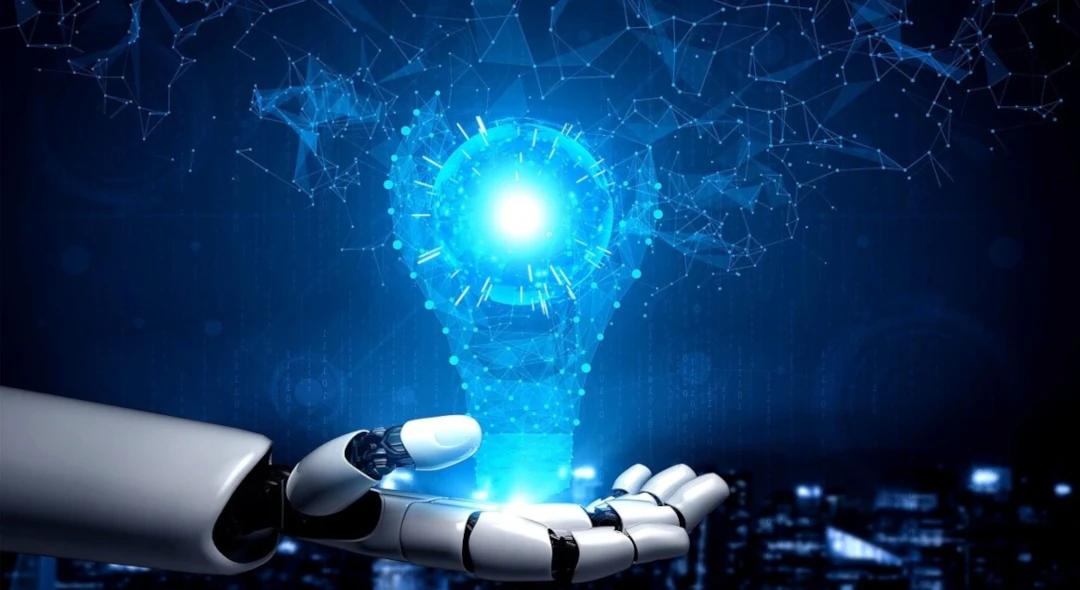
Artificial Intelligence (AI) stripes to recognize, mimic and function similar to humans. AI has currently become an integral aspect of our lives. However, to thrive in this modern digital landscape, testing is crucial for quality assurance. As a result, to keep pace with evolving trends, organizations are adopting test automation as it provides better quality, efficiency and speed of delivery of a product. Currently, achieving total automation without human intervention is not yet possible. Leveraging AI and Machine learning (ML) with test automation can benefit organizations by enabling them to automate the maximum number of tasks. This method enables test automation to incorporate AI in multiple facets which includes having an understanding of how APIs function, developing powerful API test scenarios and implementing the correct tests at the exact required time.
Let’s dive deeper and look at five key ways AI can change software automation testing:
1) Delivering Quality Product with Speed
Automation testing has already demonstrated its ability to decrease human intervention. AI further decreases it by taking on the manual work usually performed by humans. Although certain tasks like supervising, validating, analyzing machine identified anomalies, rectifying decision making, executing exploratory tests are carried out by humans and are currently not feasible for machines to perform.
AI will take over the handling of activities that are time-consuming and backbreaking. Activities like detecting iterative test cases, identifying bugs from the thousand lines of code that exist, programming regression test cases etc.
In the age of AI growth, man and machine will work together for producing efficient results. The combination of using AI and human intelligence is referred to as Intelligent Automation - it enables testers to deliver a high-quality product on time.
2) Steady Agile Testing with 24/7 Maintenance
Testing such as API testing, unit testing etc are supposed to be regularly performed. Test cases are often executed automatically in automation testing but maintaining these test cases is often a time-intensive manual task. Machine learning is capable of automating these tasks by leveraging various algorithms. AI uses stored data to gain a better understanding of the everyday behavior of test cycles. When the test cases are executed, the current state of the product is compared with the data collected, if certain changes are needed at any aspect of the execution, the test cases are amended accordingly. This enables the test cases to be maintained without requiring human intervention.
3) The Self-recovery process removes Unconventional Tasks
AI carries out a self-recovery process and improves its existing processes to become more efficient on a regular basis. Data collected from AI processes is stored regularly and can be used by the AI for self-recovery. It can anticipate future processes and accordingly mitigate risks. During the self-recovery process, AI detects and resolves the error often before it has even occurred. AI continuously collects data and updates its algorithms. This enables the detection of the behavior of the application and in delivering a product that is highly efficient.
4) Independent Tests
If tests rely on certain modules or responses for execution, it hampers automation and makes it more complicated. Earlier, pseudo responses were developed for executing successful test cases. However pseudo responses are no longer used after the introduction of AI. AI is capable of collecting and storing responses from the servers after a few manual tests have been executed. Such responses are then leveraged for removing dependencies on various modules and servers. This achieves higher test efficiency without errors.
5) Regular Study and Analysis
Data analytics will pave the path for quality management using AI and ML. Using regular observations, customer’s actions will be cataloged and become part of a process that enables the delivery of a high-quality product. Using Ml in analysis enables achieving test coverage. It compares the recorded data and offers quick feedback with lesser bugs and errors.
However, before making the decision to invest in AI and ML, you must first invest in quality testers. If an organization has the best testing resources, then using the help of AI and ML can be instrumental in delivering high-quality products. The combination of the knowledge gathered by ML and AI, and leveraging that knowledge to create actionable information can work wonders for any organization.